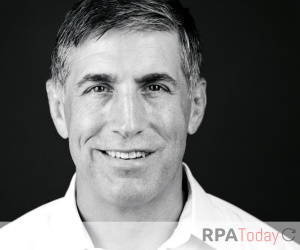
By Rik Chomko, CEO, InRule Technology
For many years, the mantra of the tech industry was to “move fast and break things.” While this approach brought us massive innovation over the past 20 years, it has also led to undesirable outcomes, such as data breaches and biased algorithms, impacting both the tech community and society at large. Those unintended consequences have resulted in a backlash against the idea of growing at all costs in favor of measured, methodical growth.
Tech leaders can no longer afford to “break things” on their quest to build the next big thing. Not breaking things, however, is easier said than done. It’s easy for businesses to get tunnel vision and not see how the solutions, processes and innovations they build may go astray and have an adverse impact on the business itself, a specific group, or the community at large. It’s necessary to put the right safeguards in place to keep teams on track, with an eye on innovating and, at the same time, leaving the world better than we found it.
Artificial Intelligence (AI) and Machine Learning (ML) have potential negative effects. When used correctly and responsibly, however, AI/ML can be a key tool helping teams stay on the right track and deliver meaningful business outcomes.
Unpacking the Mistrust of AI/ML
There are examples of when AI has gone awry. From biased decisions like Amazon’s AI recruiting tool to Google’s maybe-sentient AI, it’s not surprising that many view AI/ML through a skeptical lens. In fact, a newly commissioned research study conducted by Forrester Consulting on behalf of InRule, found that AI/ML leaders worry that AI/ML decisioning can create harmful bias which can lead to inaccurate (58 percent) or inconsistent (46 percent) decisions, decreased operational efficiency (39 percent), and loss of business (32 percent).
So where did this mistrust come from?
The crux of this mistrust – like with most things – is a misunderstanding about what exactly the purpose and use of AI is and should be. Even its name, artificial intelligence, doesn’t accurately showcase what the technology can do and how it should be used. At its core, AI is built for human empowerment. The goal isn’t to make AI systems as human as possible; rather, it is to enhance the human experience through optimized business processes or personalized (and expedient) customer experiences.
AI should be seen as uplifting what human intelligence is already capable of. Technology must work hand-in-hand with humans for it to truly empower the business processes we utilize it for. There is intrinsic value in bringing human intelligence to the AI decision making process. A reframing of how we discuss and think about AI is needed to further develop, regulate and advance how it is used in enterprises today.
Through the lens of intelligence automation, we can begin to put humans back into the lifecycle of AI. By focusing on keeping humans in the loop, we can foster greater explainability, minimize (and ideally eliminate) harmful bias in algorithms, and ultimately rebuild the trust that has eroded the credibility of AI solutions in recent years.
Using AI to Do Good – Starting Small
For businesses that are working hard to meet deadlines, deliverables and revenue goals, it might be difficult to prioritize what is right over what is easy, but the two do not have to be mutually exclusive. More often than not, doing the right thing is the easy thing, especially when you start small.
In industries like retail, healthcare or financial services there is a concerted push to digitize manual workflows and decisions, which many times results in throwing AI algorithms against the wall and seeing what sticks.
During the last few years, enterprises have accelerated digital transformation efforts out of necessity. While many organizations have implemented solutions such as RPA, DPA and automated decisoning, they may have run up against issues of maintenance and ROI. For instance, an enterprise might have adopted a solution, only to find out that it’s not delivering the intended results, that it can’t scale as planned, or that it needs more regular maintenance and updates than the organization was initially prepared to provide. Rather than focusing on digitizing and automating everything at once, businesses should start small to ensure they are staying on track and that the tech they are utilizing is operating properly and delivering intended outcomes in line with ethical and corporate governance requirements.
A Focus On Explainability Keeps AI on the Right Track
C-suite executives consistently rank AI as critically important to the future of their business, yet two-thirds of those surveyed by Forrester Consulting have difficulty explaining the decisions their AI systems make.
To foster and retain trust, organizations must deploy explainable AI solutions that provide transparency and instill confidence that outcomes are free from harmful bias. Further, explainable AI solutions make it much easier to meet current and upcoming regulatory standards related to ethics and discrimination.
However, not all AI explainability solutions and bias detection features are created equal. In fact, some solutions available today aren’t able to deliver insights as to why an algorithm delivered a specific outcome. Additionally, most machine learning platforms that offer bias detection today only pursue bias at the model’s highest, most overarching level; it’s important to seek out solutions that scour the deepest subsets of a model, exploring millions of data paths to ensure that the model operates with equal fairness within groups and between groups.
One fundamental step organizations can take to reduce the risk of harmful bias and negative outcomes from AI is to keep humans involved in the decision-making process. Human-in-the-loop AI is designed to thoughtfully include humans in the automated decisioning lifecycle. The Forrester Consulting survey found that 70 percent of decision-makers agree involving humans in decisioning with AI/ML reduces risks. Utilizing a human-in-the-loop-approach to AI enables teams to leverage human accountability within AI-powered automation to better predict customer needs, personalize solutions, and validate outcomes when they are challenged.
An explainability-focused, human-in-the-loop-approach to AI reduces the risk of harmful or biased outcomes that can be detrimental to the business or the customer. Beyond explainability and transparency, ensuring that systems can alert humans for exception handling allows for smarter decisioning, reduces mundane tasks for employees, and saves the organization time and resources that could be focused on high-level projects and tasks.
Staying on track and ensuring you are always innovating towards the north star of doing good for all, not just a select few, starts with having the right checks and balances in your tech stack. It is critical that everyone in the tech community takes a step back from their work to truly scrutinize the “why” of what they are doing to make sure we don’t get tunnel vision and truly leave the world better than we found it. AI and ML can be key tools in achieving that if the steps are taken to safeguard.
If you liked this article, please sign up to RPA Today! Registrants will receive our free weekly RPA newsletter updating you on the most recent developments in the Robotic Process Automation, Intelligent Automation and AI space. In addition to news updates, we will also provide feature articles (like this one) with a more in-depth examination of RPA issues for end users and their enterprises.